Table of Contents
Best GPU for AI/ML, deep learning, data science in 2025: RTX 4090 vs. 6000 Ada vs A5000 vs A100 benchmarks (FP32, FP16) [ Updated ]
Using deep learning benchmarks, we will be comparing the performance of the most popular GPUs for deep learning in 2024: NVIDIA's RTX 4090, RTX 4080, RTX 6000 Ada, RTX 3090, A100, H100, A6000, A5000, and A4000.
- We used TensorFlow's standard "tf_cnn_benchmarks.py" benchmark script from the official GitHub (more details).
- We ran tests on the following networks: ResNet-50, ResNet-152, Inception v3, Inception v4, VGG-16.
- We compared FP16 to FP32 performance and used maxed batch sizes for each GPU.
- We compared GPU scaling up to 4x GPUs!
Test Bench:
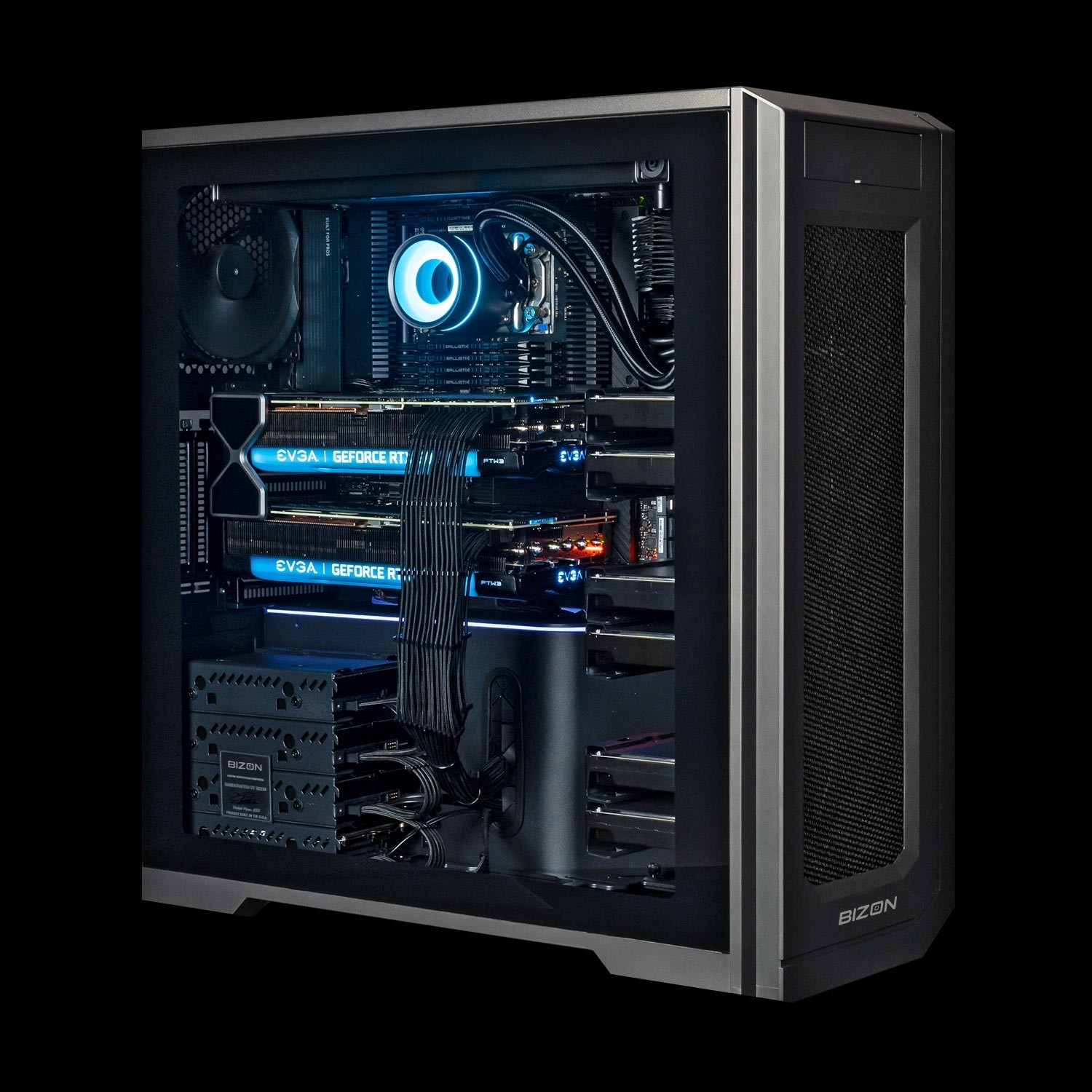
More details:https://bizon-tech.com/bizon-x5500.html
Tech specs:
- CPU: 32-Core 3.90 GHz AMD Threadripper Pro 5000WX-Series 5975WX
- Overclocking: Stage #2 +200 MHz (up to +10% performance)
- Cooling: Liquid Cooling System (CPU; extra stability and low noise)
- Memory: 256 GB (8 x 32 GB) DDR4 3200 MHz
- Operating System: BIZON Z–Stack (Ubuntu 20.04 (Bionic) with preinstalled deep learning frameworks)
- HDD: 1TB PCIe SSD
- Network: 10 GBIT
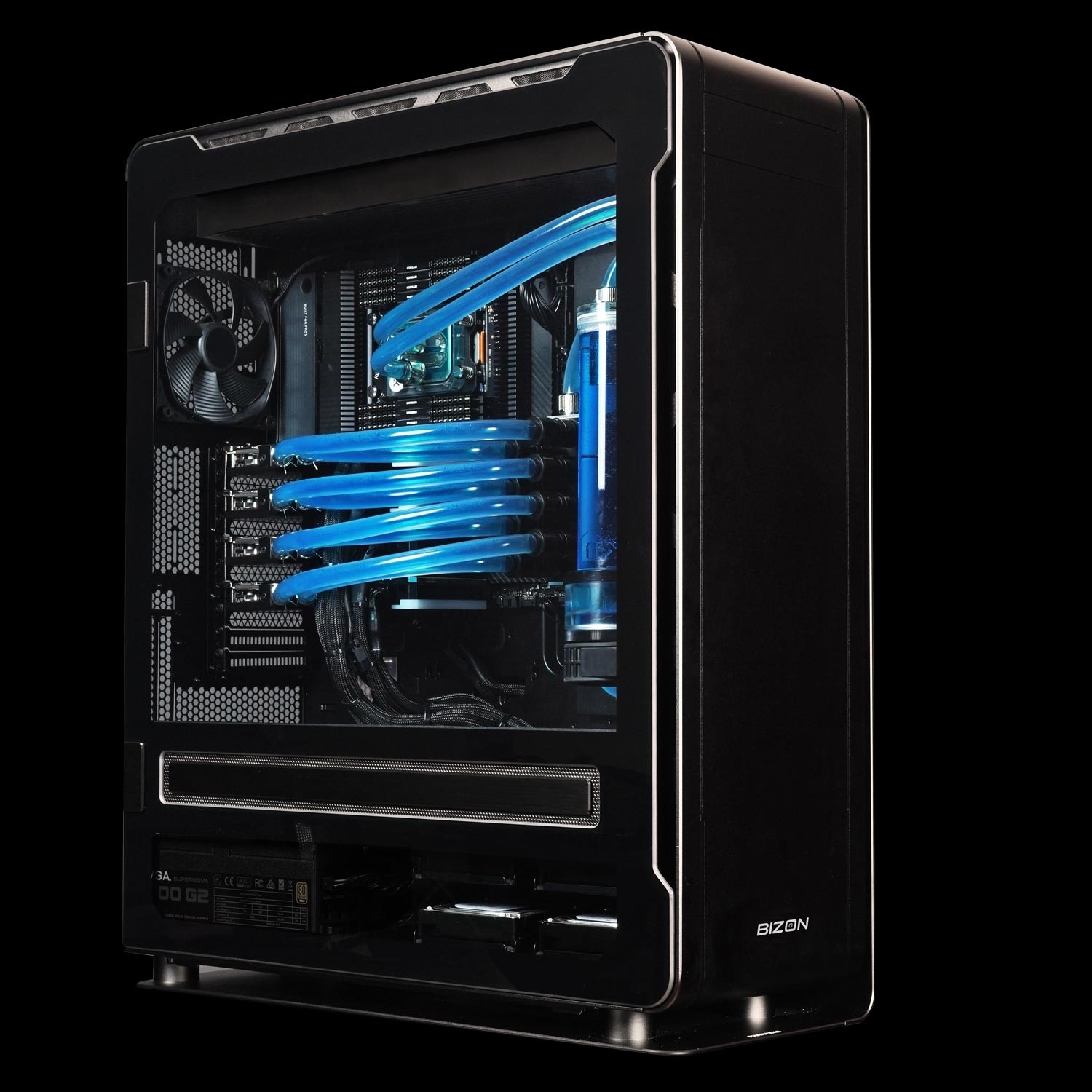
More details:https://bizon-tech.com/bizon-zx5500.html
Tech specs:
- CPU: 64-Core 3.5 GHz AMD Threadripper Pro 5995WX
- Overclocking: Stage #2 +200 MHz (up to + 10% performance)
- Cooling: Custom water-cooling system (CPU + GPUs)
- Memory: 256 GB (8 x 32 GB) DDR4 3200 MHz
- Operating System: BIZON Z–Stack (Ubuntu 20.04 (Bionic) with preinstalled deep learning frameworks)
- HDD: 1TB PCIe SSD
- Network: 10 GBIT
Deep Learning Models:
- Resnet50
- Resnet152
- Inception V3
- Inception V4
- VGG16
- Nvidia Driver: 470
- CUDA: 11.2
- TensorFlow: 2.40
Benchmarks
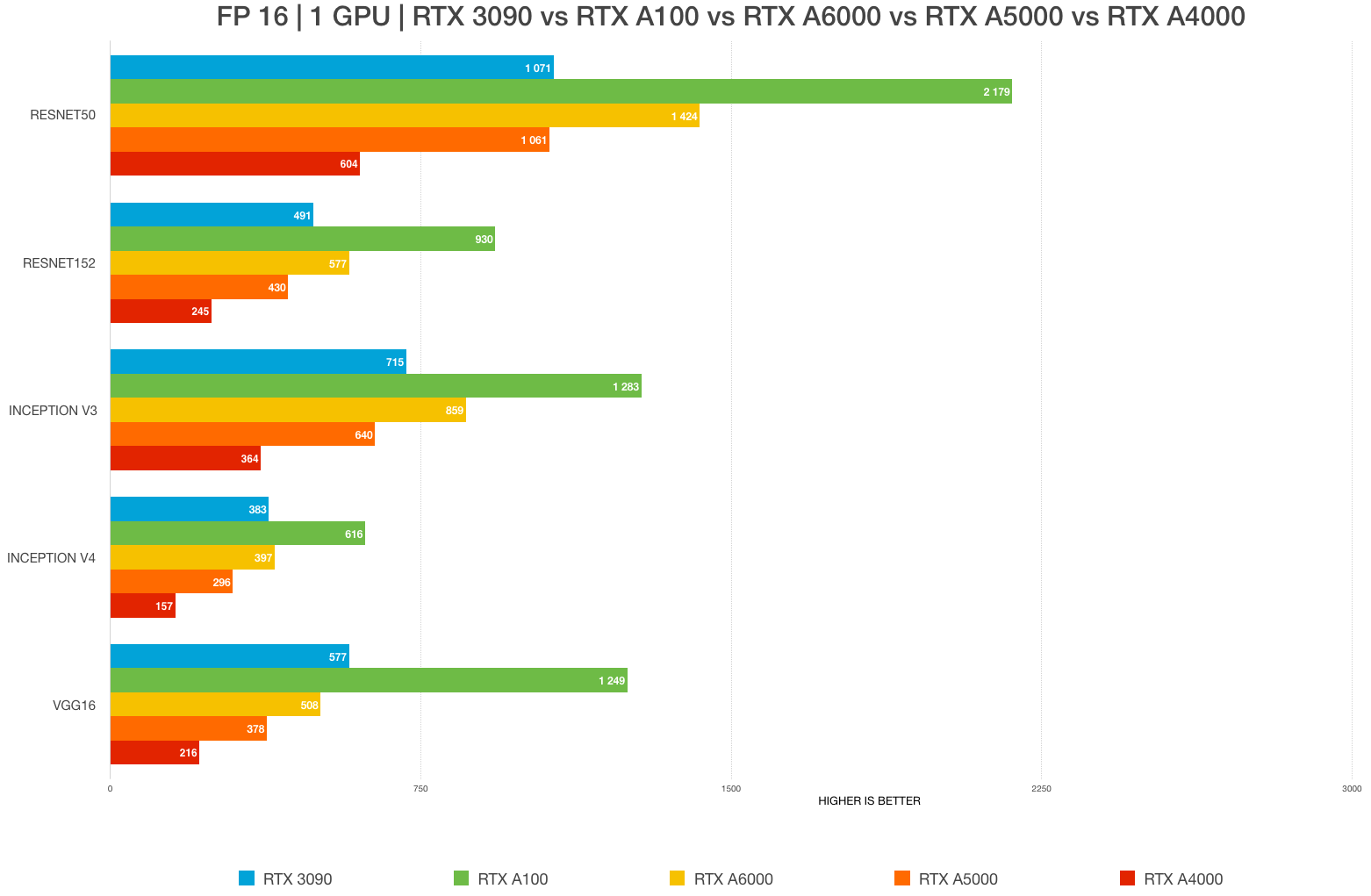
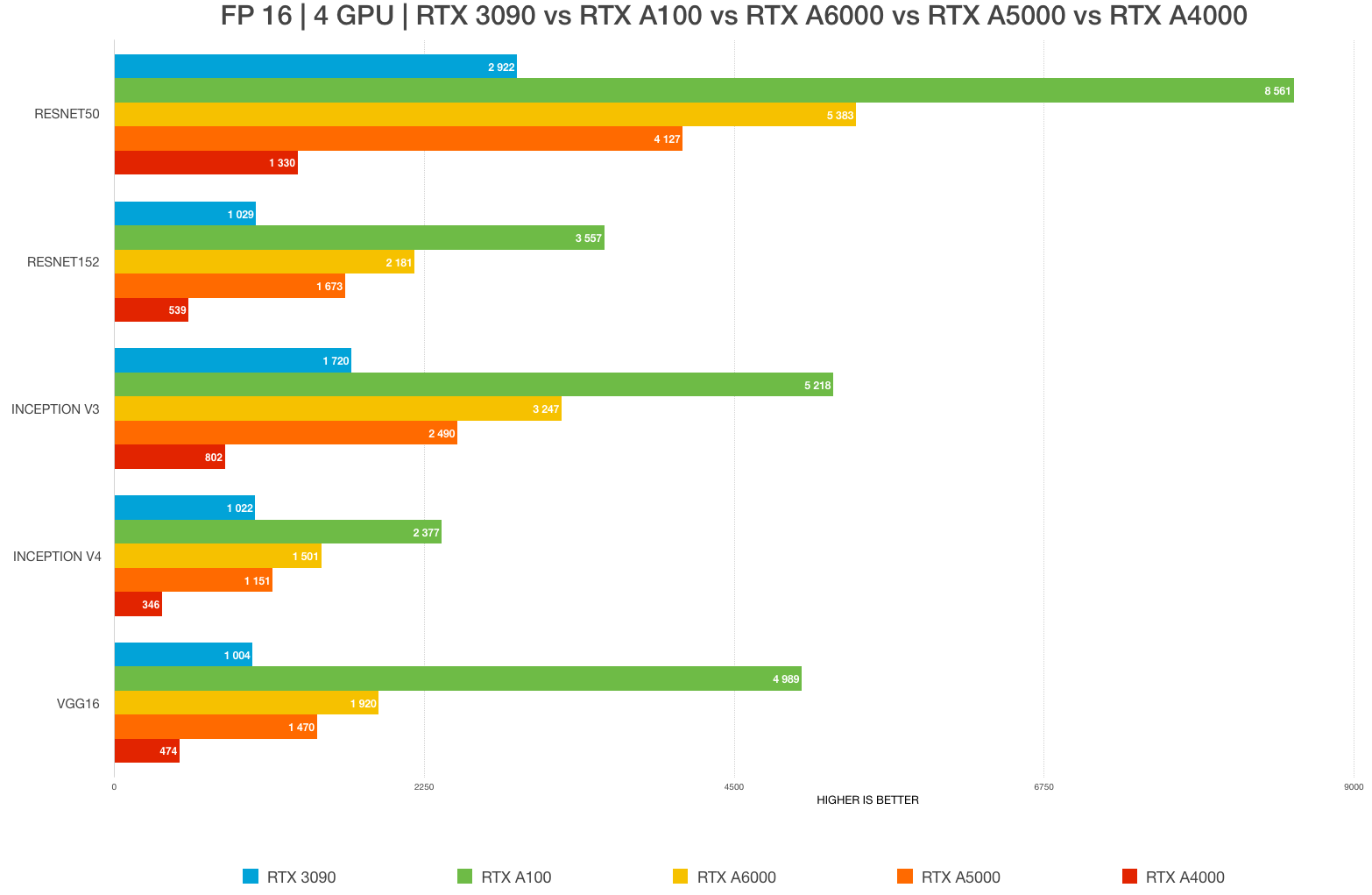
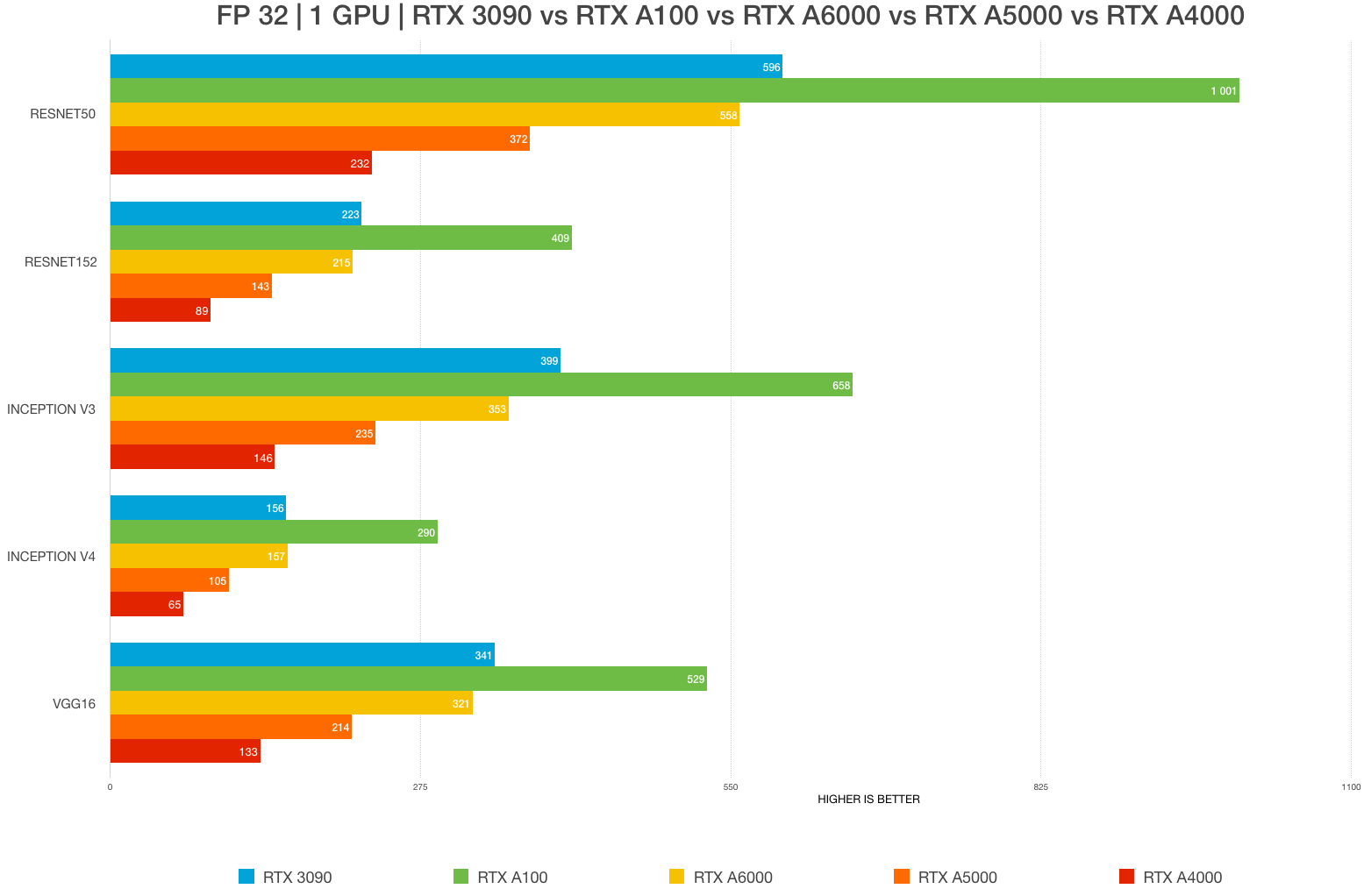
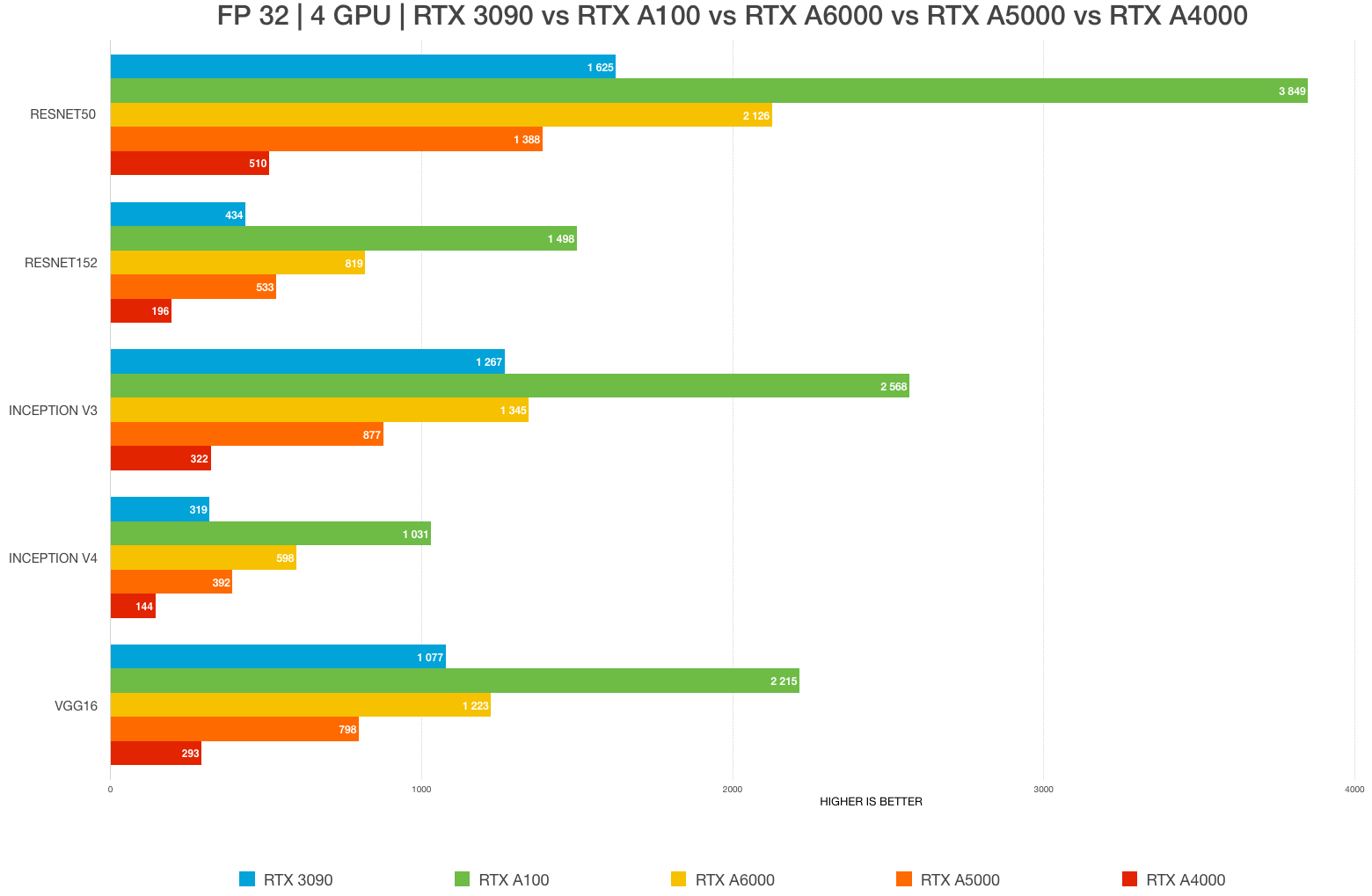
Note: Due to their 2.5 slot design, RTX 3090 GPUs can only be tested in 2-GPU configurations when air-cooled. Water-cooling is required for 4-GPU configurations.
Conclusion – Recommended hardware for deep learning, AI, and data science
Best GPU for AI in 2024 2023:NVIDIA RTX 4090, 24 GB – Price: $1599
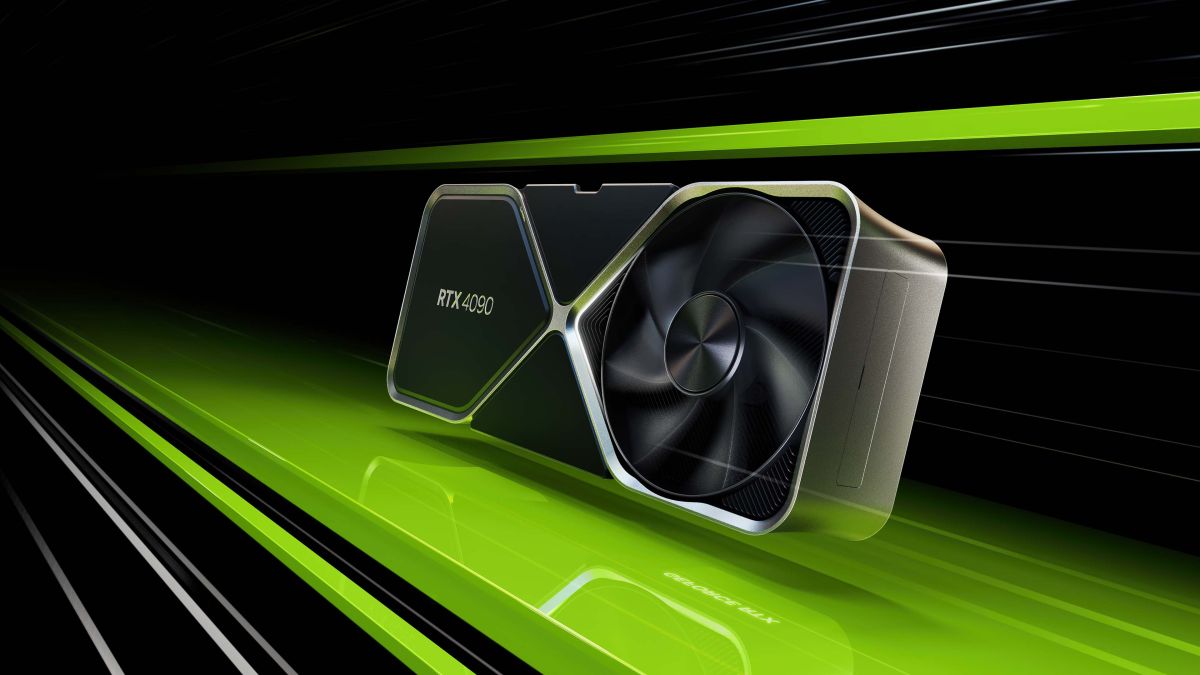
Notes: Water cooling required for 2x–4x RTX 4090 configurations.
NVIDIA's RTX 4090 is the best GPU for deep learning and AI in 2024 and 2023. It has exceptional performance and features that make it perfect for powering the latest generation of neural networks. Whether you're a data scientist, researcher, or developer, the RTX 4090 24GB will help you take your projects to the next level.
A problem some may encounter with the RTX 4090 is cooling, mainly in multi-GPU configurations. Due to its massive TDP of 450W-500W and quad-slot fan design, it will immediately activate thermal throttling and then shut off at 95°C.
We have seen an up to 60% (!) performance drop due to overheating.
Liquid cooling is the best solution, providing 24/7 stability, low noise, and greater hardware longevity. Plus, any water-cooled GPU is guaranteed to run at its maximum possible performance. Quad-slot RTX 4090 GPU design limits you up to 2x 4090 per workstation and water-cooling will allow you to get up to 4 x RTX 4090 in a single workstation.
As per our tests, a water-cooled RTX 4090 will stay within a safe range of 50-60°C vs 90°C when air-cooled (95°C is the red zone where the GPU will stop working and shutdown). Noise is another important point to mention. Air-cooled GPUs are pretty noisy. Keeping the workstation in a lab or office is impossible, not to mention servers. The noise level is so high that it’s almost impossible to carry on a conversation while they are running. Without proper hearing protection, the noise level may be too high for some to bear. Liquid cooling resolves this noise issue in desktops and servers. Noise is 20% lower than air cooling. One could place a NVIDIA-powered data science workstation or server with such massive computing power in an office or lab. BIZON has designed an enterprise-class custom water-cooling system for GPU servers and AI, data science workstations.
We offer a wide range of AI/ML-optimized, deep learning NVIDIA GPU workstations and GPU-optimized servers for AI.
- BIZON G3000 – Intel Core i9 + 4 GPU AI workstation
- BIZON X5500 – AMD Threadripper + 4 GPU AI workstation
- BIZON ZX5500 – AMD Threadripper + water-cooled 4x RTX 4090, 4080, A6000, A100
- BIZON G7000 – 8x NVIDIA GPU Server with Dual Intel Xeon Processors
- BIZON ZX9000 – Water-cooled 8x NVIDIA GPU Server with NVIDIA A100 GPUs and AMD Epyc Processors
Best GPU for AI in 2024 2023:NVIDIA RTX 4080, 12/16 GB
Price: $1199
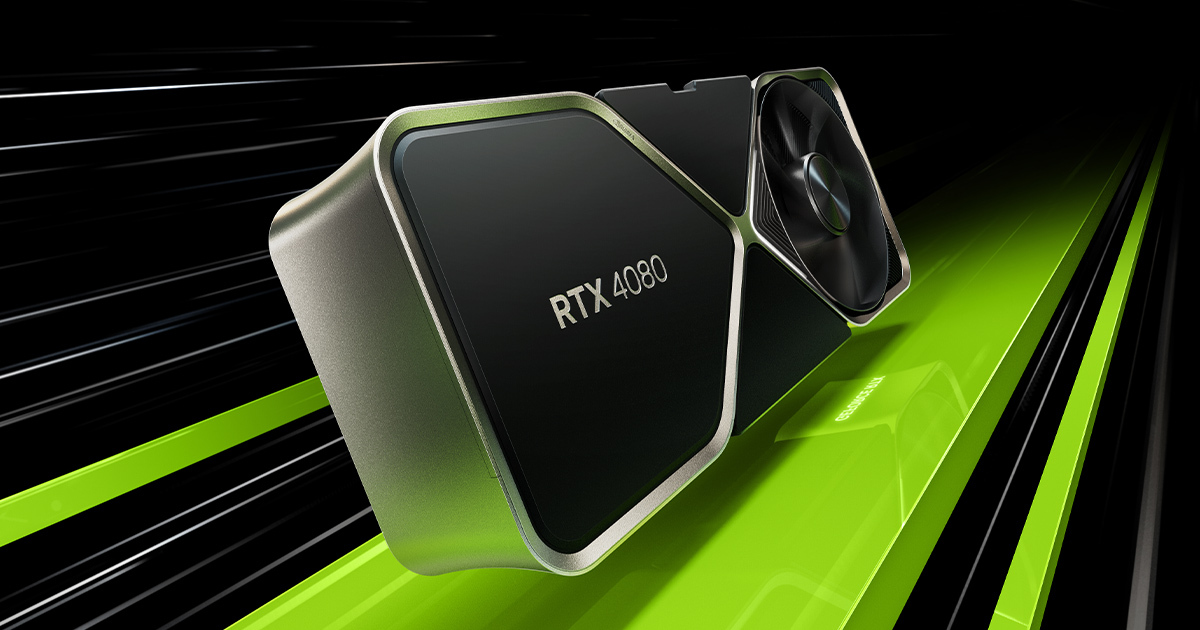
Notes: Water cooling required for 2x–4x RTX 4080 configurations.
NVIDIA RTX 4080 12GB/16GB is a powerful and efficient graphics card that delivers great AI performance. Featuring low power consumption, this card is perfect choice for customers who wants to get the most out of their systems. RTX 4080 has a triple-slot design, you can get up to 2x GPUs in a workstation PC.
We offer a wide range of deep learning NVIDIA GPU workstations and GPU optimized servers for AI.
- BIZON G3000 – Intel Core i9 + 4 GPU AI workstation
- BIZON X5500 – AMD Threadripper + 4 GPU AI workstation
- BIZON ZX5500 – AMD Threadripper + water-cooled 4x RTX 4090, 4080, A6000, A100
- BIZON G7000 – 8x NVIDIA GPU Server with Dual Intel Xeon Processors
- BIZON ZX9000 – Water-cooled 8x NVIDIA GPU Server with NVIDIA A100 GPUs and AMD Epyc Processors
Best GPU for AI in 2020 2021:NVIDIA RTX 3090, 24 GB
Price: $1599
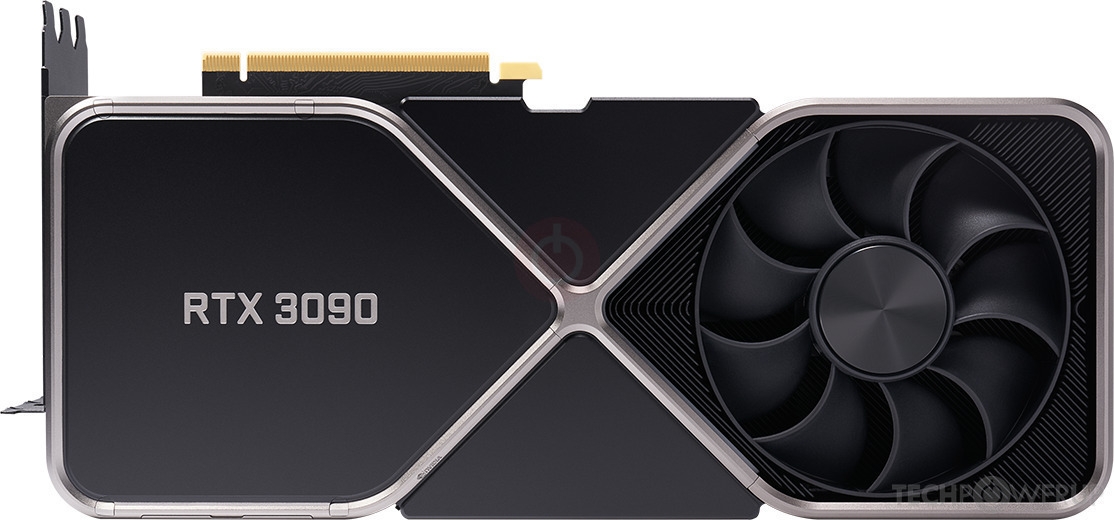
Notes: Water cooling required for 4 x RTX 3090 configurations.
NVIDIA's RTX 3090 is the best GPU for deep learning and AI in 2020 2021. It has exceptional performance and features make it perfect for powering the latest generation of neural networks. Whether you're a data scientist, researcher, or developer, the RTX 3090 will help you take your projects to the next level.
The RTX 3090 has the best of both worlds: excellent performance and price. The RTX 3090 is the only GPU model in the 30-series capable of scaling with an NVLink bridge. When used as a pair with an NVLink bridge, one effectively has 48 GB of memory to train large models. A problem some may encounter with the RTX 3090 is cooling, mainly in multi-GPU configurations. Due to its massive TDP of 350W and the RTX 3090 does not have blower-style fans, it will immediately activate thermal throttling and then shut off at 90°C.
We have seen an up to 60% (!) performance drop due to overheating.
Liquid cooling is the best solution; providing 24/7 stability, low noise, and greater hardware longevity. Plus, any water-cooled GPU is guaranteed to run at its maximum possible performance. As per our tests, a water-cooled RTX 3090 will stay within a safe range of 50-60°C vs 90°C when air-cooled (90°C is the red zone where the GPU will stop working and shutdown). Noise is another important point to mention. 2x or 4x air-cooled GPUs are pretty noisy, especially with blower-style fans. Keeping the workstation in a lab or office is impossible - not to mention servers. The noise level is so high that it’s almost impossible to carry on a conversation while they are running. Without proper hearing protection, the noise level may be too high for some to bear. Liquid cooling resolves this noise issue in desktops and servers. Noise is 20% lower than air cooling. One could place a workstation or server with such massive computing power in an office or lab. BIZON has designed an enterprise-class custom liquid-cooling system for servers and workstations.
We offer a wide range of deep learning, data science workstations and GPU-optimized servers.
Recommended AI, data science workstations:
- BIZON G3000 - Core i9 + 4 GPU AI workstation
- BIZON X5500 - AMD Threadripper + 4 GPU AI workstation
- BIZON ZX5500 - AMD Threadripper + water-cooled 4x RTX 3090, A6000, A100
Recommended NVIDIA GPU servers:
- BIZON G7000 - 8x NVIDIA GPU Server with Dual Intel Xeon Processors
- BIZON ZX9000 - Water-cooled 8x NVIDIA GPU Server with NVIDIA A100 GPUs and AMD Epyc Processors
NVIDIA A100, 80 GB
Price: $13999
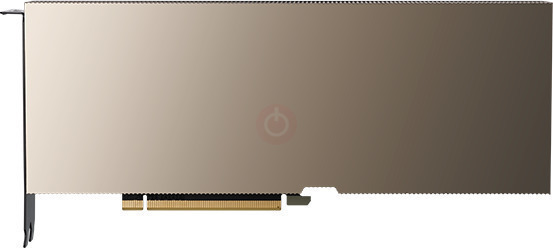
Academic discounts are available.
NVIDIA A100 is the world's most advanced deep learning accelerator. It delivers the performance and flexibility you need to build intelligent machines that can see, hear, speak, and understand your world. Powered by the latest NVIDIA Ampere architecture, the A100 delivers up to 5x more training performance than previous-generation GPUs. Plus, it supports many AI applications and frameworks, making it the perfect choice for any deep learning deployment.
We offer a wide range of deep learning workstations and GPU-optimized servers.
Recommended NVIDIA A100 workstations:
- BIZON G7000 - 8x NVIDIA GPU Server with Dual Intel Xeon Processors
- BIZON ZX9000 - Water-cooled 8x NVIDIA GPU Server with Dual AMD Epyc Processors
NVIDIA A6000, 48 GB
Price: $4650
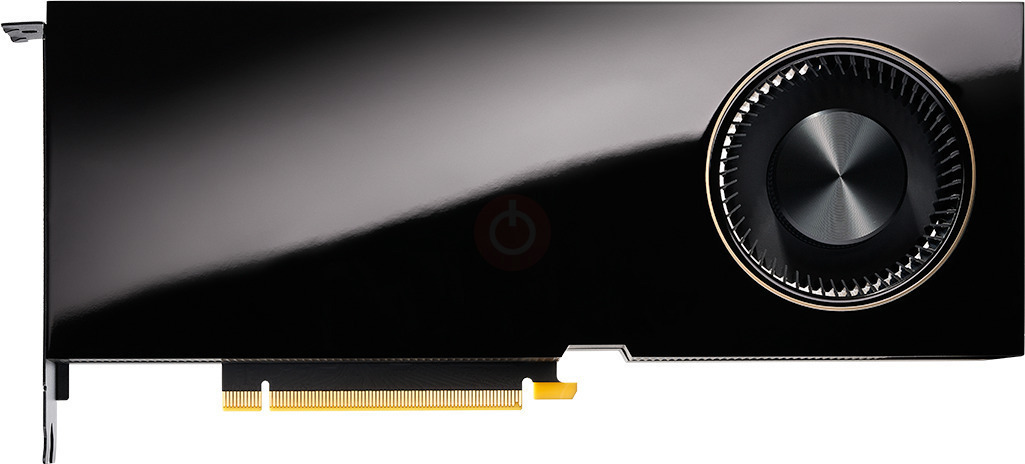
The NVIDIA A6000 GPU offers the perfect blend of performance and price, making it the ideal choice for professionals. With its advanced CUDA architecture and 48GB of GDDR6 memory, the A6000 delivers stunning performance. Training on RTX A6000 can be run with the max batch sizes.
We offer a wide range of deep learning workstations and GPU optimized servers.
Recommended NVIDIA RTX A6000 data science workstations:
- BIZON G3000 - Core i9 + 4 GPU AI workstation
- BIZON X5500 - AMD Threadripper + 4 GPU AI workstation
- BIZON ZX5500 - AMD Threadripper + water-cooled 4x RTX A6000
Recommended NVIDIA GPU servers:
- BIZON G7000 - 8x NVIDIA GPU Server with Dual Intel Xeon Processors
- BIZON ZX9000 - Water-cooled 8x NVIDIA GPU Server with Dual AMD Epyc Processors
AI Cluster Infrastructure:
NVIDIA A5000, 24 GB
Price: $3100
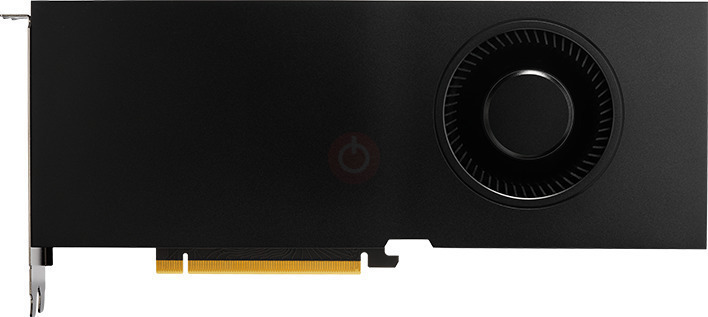
NVIDIA's A5000 GPU is the perfect balance of performance and affordability. NVIDIA A5000 can speed up your training times and improve your results. This powerful tool is perfect for data scientists, developers, and researchers who want to take their work to the next level.
We offer a wide range of AI/ML, deep learning, data science workstations and GPU-optimized servers.
Recommended AI/ML workstations:
- BIZON G3000 - Core i9 + 4 GPU AI workstation
- BIZON X5500 - AMD Threadripper + 4 GPU AI workstation
- BIZON ZX5500 - AMD Threadripper + water-cooled 4x RTX A5000
Recommended NVIDIA GPU servers:
- BIZON G7000 - 8x NVIDIA GPU Server with Dual Intel Xeon Processors
- BIZON ZX9000 - Water-cooled 8x NVIDIA GPU Server with Dual AMD Epyc Processors
NVIDIA A4000, 16 GB
Price: $1300
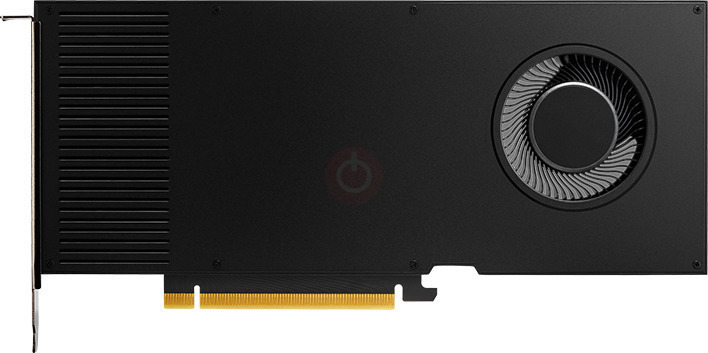
NVIDIA A4000 is a powerful and efficient graphics card that delivers great AI performance. Featuring low power consumption, this card is perfect choice for customers who wants to get the most out of their systems. RTX A4000 has a single-slot design, you can get up to 7 GPUs in a workstation PC.
We offer a wide range of deep learning workstations and GPU optimized servers.
Recommended data science workstations:
You can find more NVIDIA RTX A6000 vs RTX A5000 vs RTX A4000 vs RTX 3090 GPU Deep Learning Benchmarkshere.
For most users, NVIDIA RTX 4090, RTX 3090 or NVIDIA A5000 will provide the best bang for their buck.
Working with a large batch size allows models to train faster and more accurately, saving time.
With the latest generation, this is only possible with NVIDIA A6000 or RTX 4090.
Using FP16 allows models to fit in GPUs with insufficient VRAM.
24 GB of VRAM on the RTX 4090, and RTX 3090 is more than enough for most use cases, allowing space for almost any model and large batch size.